Join As Students, Leave As Professionals.
Develearn is the best institute in Mumbai, a perfect place to upgrade your skills and get yourself to the next level. Enroll now, grow with us and get hired.
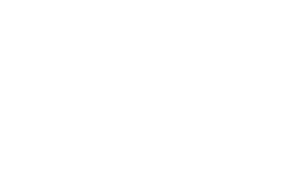
Modin: Supercharge Your Pandas Workflows with Parallel Processing
Learn about Modin, a powerful DataFrame library that harnesses the full potential of your CPU cores to accelerate pandas workflows. Discover how it seamlessly integrates with pandas, making it easy to work with large datasets and achieve significant performance improvements with just one line of code change.
Modin
Pandas
Artificial intelligence
Data science
Develearn Technologies
6 minutes
August 1, 2023
What is Modin?
Modin is a DataFrame library that wraps pandas and transparently distributes the data and computation, accelerating your pandas workflows with just one line of code change. The user does not need to know how many cores their system has, nor do they need to specify how to distribute the data through out the core. In fact, users can continue using their previous pandas while experiencing a considerable speedup from the Modin library, even on a single machine. Only a single line modification of the import statement is needed.
Sounds great, right? Lets take a deeper look at how this works today.
Modin makes user of CPU cores as compared to pandas. A machine running Modin makes use of all available cores in the system where as with pandas only one core is in use, while others often sit idle. Hence, when we deal with large datasets, the performance of every data manipulation operation is affected and can speed up the process by utilization of all cores.
Why Modin?
Modin is a tool which exactly satisfies the above phrase which basically allows us to work with large data frames. Pandas does not have multiprocessing support and it is slow with bigger datasets(1MB to1TB+). With Pandas, by default we can only use a single CPU core at a time. This is usually fine for small datasets, but when working with larger(& often real-world) files this can create a bottleneck issue. For smaller datasets you might not notice much of a difference in speed. But with larger datasets, and so many more calculations, speed factors start to take a major hit when using only a single core and this can affect overall performance. It is doing just one calculation at a time for a dataset that can have millions or even billions of rows with the single core. There is a better option that puts those CPU cores to work!
Checking the number of cores in your PC:
You can check the number of cores your system uses by checking the Hardware properties of your system. The image above shows a sample for Apple devices but the location is correspondingly similar for Windows PCs.
How Modin does Parallel Processing with Pandas:
Modin slices your Data Frame into different parts such that each part can be sent to a different CPU core. Modin partitions the Data Frames across both the rows and the columns. This makes Modin’s parallel processing scalable to Data Frames of any shape. As shown below, a modin data frame works by dividing across rows and columns whereas pandas works on data frames without any division across rows or columns and hence, are much slower.
Modin:Setup and Implementation
Modin provides a pandas-like API that uses Ray or Dask to implement a high-performance distributed execution framework to work with larger dataset. With Modin you can use all the CPU cores on your machine. It provides speed-ups of up to 4x on a laptop with 4 physical cores [Modin Docs].
Installation:
Installing with pip and Stable version
Modin can be installed with pip. To install the most recent stable release run the following:
pip install -U modin
# -U for upgrade in case you have an older version
If you don’t have Ray or Dask installed, you will need to install Modin with one of the below.
# Install Modin dependencies and Ray to run on Ray
pip install modin[ray]# Install Modin dependencies and Dask to run on Dask
pip install modin[dask]# Install all of the above
pip install modin[all]
Modin will automatically detect which engine you have installed and use that for scheduling computation!
Windows installation
For installation on Windows,recommended to use Dask Engine. Ray does not support Windows, so it will not be possible to install modin[ray] or modin[all]. It is possible to use Windows Subsystem For Linux (WSL), but this is generally not recommended due to the limitations and poor performance of Ray on WSL, a roughly 2–3x cost. To install with the Dask engine, run the following using pip:
pip install modin[dask]
if you already have a recent version of Dask installed, then you can simply type pip install modin.
How to use Modin: Scale your pandas workflow by changing a single line of code
Modin uses either Ray or Dask to provide an effortless way to speed up your pandas data frame,, scripts, and libraries. Unlike other distributed DataFrame libraries, Modin provides seamless integration and compatibility with existing pandas code.
import modin.pandas as pd
import numpy as npframe_data=np.random.randint(0,100,size=(2**10,2**8))
df=pd.DataFrame(frame_data)
Benchmark 1: READ a CSV file
Let’s go ahead and read a 3gigabytes CSV file using Pandas, cuDF and Modin. We’ll run it 30 times and get the mean values.
Sample code for reading data:
import pandas as pd
import modin.pandas as pd_modin
import cudf as pd_cudfresults_loading = []### Read in the data with Pandasfor run in range(0,30):
s = time.time()
df = pd.read_csv(“CITY.CSV”)
e = time.time()
results_loading.append({“lib”:”Pandas”,”time”:float(“{
}”,format(e-s))})print(“Pandas Loading Time = {}”.format(e-s))### Read in the data with Modinfor run in range(0,30):
s = time.time()
df = pd_modin.read_csv(“CITY.CSV”)
e = time.time()
results_loading.append({“lib”:”Modin”,”time”:float(“{
}”.format(e-s))})print(“Modin Loading Time = {}”.format(e-s))### Read in the data with cudffor run in range(0,30):
s = time.time()
df = pd_cudf.read_csv(“CITY.CSV”)
e = time.time()
results_loading.append({“lib”:”Cudf”,”time”:float(“{
}”.format(e-s))})print(“Cudf Loading Time = {}”.format(e-s))
READ a CSV file benchmark:
Missing values benchmark:
GROUP BY clause benchmark:
So, which library is the best?
lets lay out the context of each library
Modin, with Ray as a back-end library gets significant benefits by changing just a single line (`import pandas as pd` to import modin.pandas as pd
). Modin is fully compatible with pandas.
Dask: Has a slightly more complicated usage, but is also a higher-level Pandas-like library that can help to deal with data sets.
Vaex: Designed to help you work with large data on a standard laptop. Its Pandas replacement covers some of the Pandas API, but it’s more focused on exploration and visualization and also has complicated usage .
RAPIDS: A great library with rapid performance, but strictly requires a NVIDIA graphics cards
Which library should one use?
In today’s benchmark, reading the file was much faster using Modin, but how many times do we need to use the read_csv()
method in our ETL? By contrast, in theory, we would use the groupby()
method more frequently, and in this case, the cuDF library had the best performance.
Modin is pretty easy to install (we just need to use pip) and cuDF is relatively harder (you’ll need to update your NVIDIA drivers, install CUDA and then install cuDF using conda in that order), or you can skip all these steps and get a PC optimised for Data Science as it would comes with all RAPIDS libraries and software fully installed.
Also, both Modin and cuDF are still in the early stages and they don’t have the complete coverage of the entire Pandas API yet. Nevertheless, modin will certainly grow to become an essential tool in any Data Analyst’s toolkit.