Join As Students, Leave As Professionals.
Develearn is the best institute in Mumbai, a perfect place to upgrade your skills and get yourself to the next level. Enroll now, grow with us and get hired.
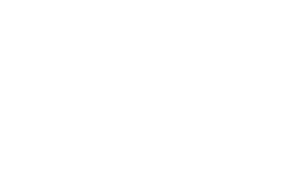
What is Data Science: Lifecycle, Techniques, Eligibility Criteria and Tools
Discover the world of data science, from its lifecycle and techniques to the key eligibility criteria for aspiring professionals. Explore essential tools used in data science to harness the power of data for informed decision-making.
DeveLearn Technologies
30 minutes
March 20, 2024
What Is Data Science?
Data science is a field of study that combines various disciplines to extract valuable information and useful insights from complex data sets. It involves areas such as mathematics, statistics, computer science, and artificial intelligence, and it is widely applied across different sectors to improve planning and decision-making processes.
Data science professionals, known as data scientists, utilize artificial intelligence, particularly machine learning and deep learning, to develop models and generate predictions through advanced algorithms and methods.
DATA SCIENCE DEFINITION: BASICS OF DATA SCIENCE
What Is Data Science Used for?
Data science is utilized by businesses ranging from large corporations to startups to uncover correlations, patterns, and valuable insights, leading to groundbreaking discoveries. This explains the rapid growth of data science, which is transforming numerous industries. Specifically, data science is applied for intricate data analysis, predictive modeling, recommendation generation, and data visualization.
Complex Data Analysis
Data science facilitates swift and accurate analysis. Equipped with diverse software tools and methodologies, data analysts can efficiently identify trends and patterns within extensive and intricate datasets. This empowers businesses to make informed decisions, whether it involves optimizing customer segmentation or conducting comprehensive market analysis.
Predictive Modeling
Data science enables predictive modeling by identifying data patterns through machine learning techniques. Analysts can anticipate future outcomes with a certain level of accuracy, which proves beneficial in sectors like insurance, marketing, healthcare, and finance where predicting event probabilities is crucial for business success.
Recommendation Generation
Companies like Netflix, Amazon, and Spotify leverage data science and big data to generate personalized recommendations based on user behavior. Data science ensures that users receive content tailored to their preferences and interests, enhancing their overall experience on these platforms.
Data Visualization
Data science contributes to creating data visualizations such as graphs, charts, and dashboards, along with comprehensive reporting. This aids non-technical business leaders and busy executives in comprehending complex information about their business's status easily.
Data Science Tools
Data science professionals rely on a range of tools and programming languages throughout their careers. Here are some popular options used in the field today:
Common Data Science Programming Languages:
Python
R
SQL
C/C++
Popular Data Science Tools:
Apache Spark (data analytics tool)
Apache Hadoop (big data tool)
KNIME (data analytics tool)
Microsoft Excel (data analytics tool)
Microsoft Power BI (business intelligence data analytics and visualization tool)
MongoDB (database tool)
Qlik (data analytics and integration tool)
QlikView (data visualization tool)
SAS (data analytics tool)
Scikit Learn (machine learning tool)
Tableau (data visualization tool)
TensorFlow (machine learning tool)
Data Science Lifecycle
Data science follows a structured lifecycle consisting of five stages:
Capture
In this stage, data scientists gather raw and unstructured data. It involves data acquisition, data entry, signal reception, and data extraction.
Maintain
Data is transformed into a usable format during this stage. Activities include data warehousing, data cleansing, data staging, data processing, and data architecture.
Process
Data is analyzed for patterns and biases to assess its suitability for predictive analysis. This stage includes data mining, clustering, classification, data modeling, and data summarization.
Analyze
Various types of analyses are conducted on the data in this stage. It encompasses data reporting, data visualization, business intelligence, and decision-making processes.
Communicate
Data scientists and analysts present findings through reports, charts, and graphs. This stage involves exploratory and confirmatory analysis, predictive analysis, regression, text mining, and qualitative analysis.
What are the data science techniques?
Data science professionals are expected to be proficient in various techniques to effectively perform their roles. Here are some of the most popular data science techniques:
Regression
Regression analysis, a form of supervised learning, enables prediction of outcomes based on multiple variables and their interrelationships. Linear regression is the widely used technique within regression analysis.
Classification
Classification involves predicting the category or label of data points, making it another subcategory of supervised learning. Applications include email spam filters and sentiment analysis.
Clustering
Clustering, or cluster analysis, falls under unsupervised learning. It involves grouping closely related objects within a dataset and assigning characteristics to each group. Clustering is used to uncover patterns in large, unstructured datasets.
Anomaly Detection
Anomaly detection, also known as outlier detection, identifies data points with unusually extreme values. This technique is applied in industries such as finance and cybersecurity for identifying irregularities and potential threats.
Data Science Compared to Other Data Fields
Data science is a broad term that encompasses several related data fields. Let's explore the differences between data science and some of these fields:
Data Science vs. Data Analytics
Data analytics is a subset of data science, focusing primarily on statistical analysis and deriving insights from data. Data science, on the other hand, covers a broader spectrum including data collection, preprocessing, modeling, and interpretation. Data scientists may develop new methods and tools, while data analysts focus on analyzing existing data and generating reports.
Data Science vs. Business Analytics
While there's overlap, the main difference lies in technology utilization. Data scientists work more with data technology, creating models and algorithms. Business analysts bridge the gap between business and IT, defining business cases and validating solutions.
Data Science vs. Data Engineering
Data engineers build and maintain systems for data access and processing, focusing on data infrastructure. Data scientists utilize the processed data to build predictive models and gain insights.
Data Science vs. Machine Learning
Machine learning is a subset of data science, focusing on training machines to learn from data. Data scientists may use machine learning methods as tools within their projects or collaborate with machine learning engineers.
Data Science vs. Statistics
Statistics is a mathematical field focused on quantitative data analysis, while data science is multidisciplinary, incorporating scientific methods to extract knowledge from data in various forms. Data scientists often use statistical methods but also draw from other disciplines.
Data Science Careers
Data science encompasses various roles that evolve as professionals progress in their careers. Here are some common roles within data science:
Data Scientist
Data scientists focus on collecting, organizing, and analyzing data to extract actionable insights and convey them as compelling stories. They excel in uncovering patterns within large datasets using advanced algorithms and machine learning models to support accurate assessments and predictions. Data scientists typically possess strong math and statistics skills, along with proficiency in programming languages like R, Python, and SQL.
Data Analyst
Data analysts delve into datasets to find valuable insights, interpret data, and create reports, dashboards, and visualizations for internal stakeholders and sometimes external clients. They work with tools such as Tableau and Microsoft Power BI but generally don't delve into advanced statistical modeling, algorithm writing, or prediction making like data scientists do.
Learn More - Data Analyst vs. Data Scientist
Data Engineer
Data engineers design, build, and manage systems that data scientists utilize for data access and analysis. Their responsibilities include developing data models, creating data pipelines, and overseeing ETL (extract, transform, load) processes.
Business Intelligence Analyst
Business intelligence analysts specialize in analyzing data related to a company's performance to drive better business decisions. They communicate insights across teams, helping organizations understand goals and risks. BI analysts bridge the gap between non-technical stakeholders and technical data scientists within the company.
Data Science Skills
The skills and tools required for data science professionals vary depending on their specific roles and specializations within the field. However, there are general proficiencies and key soft skills that aspiring and early-career data science professionals should develop:
Programming:
Proficiency in languages like Python and R is essential for data manipulation, analysis, and modeling.
Database Management:
Learning and applying SQL is crucial for efficient communication with databases and retrieving necessary data.
Statistics:
Understanding statistical methods and techniques is vital for analyzing data and solving complex problems.
Curiosity:
Having a curious mindset helps in exploring data, identifying patterns, and continuously learning new concepts and technologies.
Storytelling:
The ability to craft compelling narratives with data and communicate insights effectively is valuable for decision-making and stakeholder engagement.
Communication:
Being comfortable collaborating with team members, conveying problems and solutions clearly, and engaging in productive discussions is essential for successful data science projects.
What is data scientist eligibility?
To qualify for a career as a data scientist, you typically need a bachelor's degree in fields like computer science, statistics, mathematics, or engineering.
Some roles may require a master's or Ph.D. Additionally, essential skills include proficiency in programming languages like Python and R, strong analytical abilities, knowledge of statistical methods and machine learning algorithms, and excellent communication skills. Practical experience through internships or projects is beneficial, along with familiarity with data science tools and technologies.
Read more about - Data Science Course Eligibility Criteria
What does a data scientist do?
A data scientist plays a crucial role in analyzing and interpreting data to extract valuable insights and make informed decisions. They use various techniques, tools, and technologies to process and analyze data efficiently. Their responsibilities and daily tasks can vary depending on the organization's size and needs.
In larger teams, data scientists collaborate with other experts such as analysts, engineers, and statisticians to ensure a comprehensive data science process. This involves selecting the best methods and technologies to achieve accurate and timely results aligned with business objectives.
In smaller teams, data scientists may take on multiple roles, combining tasks like data engineering, analysis, and machine learning. Their responsibilities can include data processing, model building, and generating actionable insights from data sets, leveraging their skills and expertise in data science methodologies.
What are the challenges faced by data scientists?
Data scientists face several challenges in their work:
Managing Multiple Data Sources:
Data scientists often deal with data from various sources in different formats. Cleaning and preparing this data to ensure consistency can be time-consuming and complex.
Defining Business Problems:
Collaborating with stakeholders and understanding their requirements to define the problem accurately can be challenging, especially in large organizations with diverse teams.
Addressing Bias in Machine Learning:
Machine learning models can exhibit biases due to imbalances in training data or prediction behavior across different groups. Detecting and mitigating biases is crucial to ensure fair and accurate predictions.
Data Privacy and Security:
Ensuring the privacy and security of sensitive data while working with large datasets is a significant concern for data scientists, requiring adherence to strict regulations and best practices.
Scalability and Performance:
Scaling data science solutions to handle large volumes of data and optimizing performance to deliver timely and accurate results are ongoing challenges faced by data scientists.
Interpreting Complex Results:
Communicating complex technical findings and insights to non-technical stakeholders in a clear and understandable manner is essential but can be difficult due to the complexity of data science processes and results.
How to become a data scientist?
Earn a bachelor's degree in IT, computer science, math, physics, or another related field.
Earn a master's degree in data science or related field.
Gain experience in a field of interest.
To further your career in data science, consider enrolling in a reputable training program. Develearn is a leading Data Science institute in Mumbai that offers comprehensive Data Science courses designed to equip individuals with the skills and knowledge needed for success in this field.
Our Data Science course in Mumbai covers a wide range of topics, including data analysis, machine learning, statistical modeling, data visualization, and more. The curriculum is crafted by industry experts and includes hands-on projects and practical training to ensure a deep understanding of key concepts and techniques.
We provides a conducive learning environment with experienced instructors, state-of-the-art facilities, and access to real-world datasets. Upon completion of the course, participants receive a certification that is recognized by industry employers, enhancing their job prospects and career advancement opportunities in the field of data science.
By joining Develearn's Data Science course in Mumbai with placement assistance, you can gain valuable skills, expand your professional network, and take significant strides towards a successful career in data science.